Overview and Uniqueness
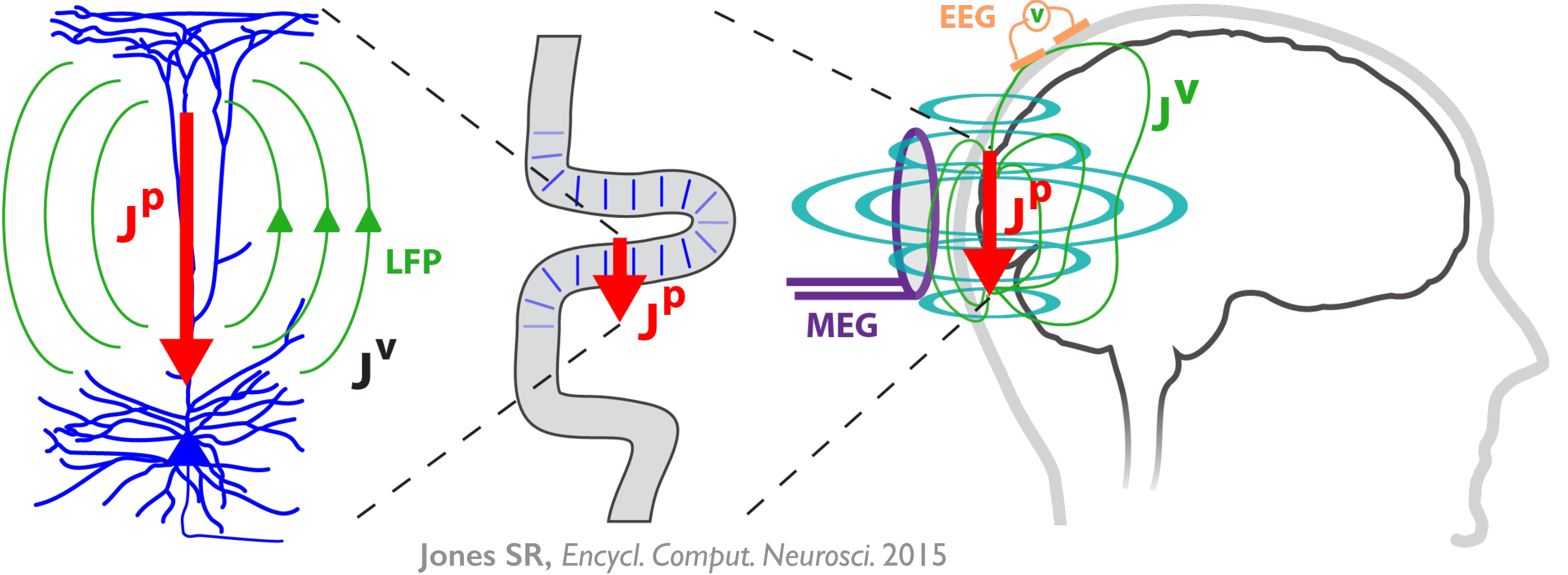
HNN is designed to be a user-friendly software tool for researchers and clinicians to develop and test hypotheses on the neural origins of their EEG/MEG data. The purpose is to bridge the “macroscale” extracranial recordings to the underlying cellular- and circuit- level activity.
HNN’s underlying neural model simulates the primary electrical currents in the neocortex that create EEG/MEG signals. The model simulations are based on the biophysical origins of the primary electrical currents (see Figure). The primary electrical currents (JP) are assumed to be generated by the post-synaptic, intracellular longitudinal current flow in the long and spatially aligned dendrites of a large population of synchronous neocortical pyramidal neurons1,2. These primary currents create volume currents (JV) that propagate through the brain tissue, cerebral spinal fluid, and skull. It is the volume currents that are pick up by the EEG/MEG sensors. “Inverse solution methods” (i.e., source localization methods) applied to the sensor data estimate the location, timing, and directionality of the primary electrical current dipole sources (Jp) (i.e., equivalent current dipoles1).
HNN simulates these primary currents from a canonical model of a layered neocortical column via the net intracellular electrical current flow in the pyramidal neuron dendrites (see schematic to the right. Further details can be found at our Under the Hood page). The units of measure that come out of the model are directly comparable to those estimated from source localization methods, namely, nano-ampere-meters (nAm). |
![]() |
A template circuit that represents a generalizable, canonical cortical column is provided. The template circuit includes a scalable number of neurons and adjustable parameters along with simulation tools to perturb the local network with layer- and cell-specific external drive (i.e., spike driven synaptic input and/or tonic applied currents). See details in Under the Hood. Tools are also provided for users to directly compare simulated electrical sources with recorded source localized data*. In future developments, we plan to integrate with source localization software (see https://www.martinos.org/mne/stable/index.html).
Importantly, HNN was created to be a hypothesis development and testing tool. While the underlying neural model is biophysically principled and based on generalizable features of cortical networks, and also includes neurons with realistic physiology and morphology (see details in Under the Hood), the model is nonetheless a reduced model of neocortical network dynamics. Any conclusions made are based on the underlying model assumptions, which are outlined in detail in our Under the Hood page. Users should become familiar with these assumptions at the start of using HNN. We have found the model to be useful for generating novel and testable hypotheses on the circuit origin of some of the most commonly measured signals, including ERPs and low-frequency oscillations3-7, which provide the basis for the tutorials. In future developments, we will create a framework for building your own cells and circuits into the software (http://www.neurosimlab.com/netpyne). We are open-source and encourage input and expansion from the community (see Users Forum).
*If you only have sensor data, you can still benefit from our software. The primary currents are the foundation of the sensor signal and, as such, will have similar activity profiles.